In re Diisocyanates Antitrust Litig.
In re Diisocyanates Antitrust Litig.
2021 WL 4295729 (W.D. Pa. 2021)
August 23, 2021
Francis IV, James C. (Ret.), Special Master
Summary
The parties disagreed over the appropriate method for estimating recall in a technology-assisted review of ESI. The court denied both the plaintiffs' motion to compel the defendants to use certain search terms and TAR methodologies, and the defendants' motion for a protective order allowing them to use their own search terms. The court recommended alternative TAR methodologies that the defendants could utilize that are reasonable.
Additional Decisions
IN RE DIISOCYANATES ANTITRUST LITIGATION
This document relates to: All Actions
This document relates to: All Actions
Master Docket Misc. No. 18-1001 | MDL No. 2862
United States District Court, W.D. Pennsylvania
Filed August 23, 2021
Francis IV, James C. (Ret.), Special Master
Report and Recommendation
*1 This multidistrict litigation involves allegations that the defendants conspired to reduce supply and increase prices for methylene diphenyl diisocyanate (“MDI”) and toluene diisocyanate (“TDI”), chemicals used in the manufacture of polyurethane foam and thermoplastic polyurethanes. The plaintiffs have moved pursuant to Rules 26 and 37 of the Federal Rules of Civil Procedure to compel defendants BASF Corporation, Covestro LLC, the Dow Chemical Company, Huntsman Corporation, and Wanhua Chemical (America) Co., Ltd. (collectively, the “defendants”)[1] to use certain search terms and technology-assisted review methodologies to identify electronically stored information (“ESI”) for production in response to the plaintiffs’ discovery requests. (ECF 455). These defendants have opposed the plaintiffs’ motion and have cross-moved pursuant to Rule 26(c) for entry of a protective order permitting the defendants to use the search terms and TAR methodologies that they have proposed to search for and review ESI. (ECF 470).
To facilitate resolution, the Court appointed me as E-Discovery Special Master pursuant to Rule 53 to submit a report and recommendation with respect to these motions. (ECF 504). I convened a preliminary conference, conducted a hearing in which technical presentations were provided by the parties’ respective experts, and heard oral argument on the motions. In addition, the parties engaged in mediation in an attempt to resolve the issues presented by the motions, but those efforts were unsuccessful. Accordingly, the motions are ripe for determination. I will describe basic aspects of technology-assisted review, set forth the parties’ competing methodologies, discuss the governing legal principles, and then apply those principles to the issues in dispute.
As will be seen, the defendants’ motion should be denied because their methodologies are not reasonable in certain critical respects. At the same time, the plaintiffs’ motion should also be denied because the Court should not foreclose the defendants from choosing alternative methodologies as long as they are reasonable.
Technology-Assisted Review
Technology-assisted review, or “TAR,”
is a process for ranking – from most to least likely to be responsive – or for classifying – as responsive or nonresponsive – a document collection, using computer software that learns to distinguish between responsive and non-responsive documents based on coding decisions made by one or more knowledgeable reviewers on a subset of the document collection. The software then applies what it has learned to the remaining documents in the collection.
(Declaration of Maura R. Grossman dated March 25, 2021 (ECF 459) (“Grossman Decl.”), ¶ 9); see also The Sedona Conference Glossary: E-Discovery & Digital Information Management (Fourth Edition), 15 Sedona Conf. J. 305, 357 (2014) (defining TAR as “[a] process for prioritizing or coding a collection of [ESI] using a computerized system that harnesses human judgments of subject matter expert(s) on a smaller set of documents and then extrapolates those judgments to the remaining documents in the collection”). TAR is often referred to as “predictive coding” because the software predicts the classification that a human reviewer would ultimately assign to a document. TAR software is also variously referred to as the TAR tool, classifier, or algorithm.
*2 It is useful to distinguish between two broad categories of TAR technology. Initially, most TAR tools relied on “seed sets” of documents to train the software. Once the software had reached a point where it would not benefit materially from additional training, it would be applied to the set of unreviewed documents and score each document for likely responsiveness. (Declaration of Daniel L. Regard II dated April 9, 2021 (ECF 471-2) (“Regard Decl.”), ¶ 26(a)); see also Bolch Judicial Inst. & Duke Law, Technology Assisted Review (TAR) Guidelines 4 (Jan. 2019) (hereinafter “Duke TAR Guidelines”), available at https://judicialstudies.duke.edu/wp-content/uploads/2019/02/TAR-Guidelines-Final-1.pdf, at 4; Lawson v. Spirit AeroSystems, Inc., No. 18-1100, 2020 WL 6343292, at *3 (D. Kan. Oct. 29, 2020). This methodology is referred to as TAR 1.0.
By contrast,
[i]n TAR 2.0, a more recent iteration of TAR technology, a classifier is continuously trained in near real-time using the documents that are coded by reviewers. This workflow is also called Continuous Active Learning (“CAL”). Training begins immediately and the classifier improves over time as more documents are reviewed. As with TAR 1.0, the TAR 2.0 classifier assigns a score to each document predicting the likelihood that it is responsive, but unlike TAR 1.0 the classifier adjusts the scores throughout the document review as it learns more. Typically, a TAR 2.0 system prioritizes documents with the highest scores (i.e., the unreviewed documents that TAR predicts are most likely to be responsive) for review. This means that once the TAR classifier has learned enough to score documents, most documents reviewed in the early stage of the review are ranked highly responsive, and there are more of them, and as the review continues, fewer and fewer responsive documents are indicated, and their ranking is lower and lower.
(Regard Decl., ¶ 26(b)(i), (ii)); see also Duke TAR Guidelines at 5; Lawson, 2020 WL 6343292, at *3; In re Valsartan, Losartan, and Irbesartan Products Liability Litigation, 337 F.R.D. 610, 614 (D.N.J. 2020).
In measuring the effectiveness of search and review, two metrics are important.
One is referred to as recall, which is a measure of completeness, reflected by the proportion (i.e., percent) of responsive documents in a collection that have been found through a search or review process, out of all possible responsive documents in the collection.... The other metric is referred to as, precision, which is a measure of accuracy, or the proportion (i.e., percent) of the documents identified by the search or review process that are actually responsive.... High recall suggests that substantially all responsive documents have been found; high precision suggests that primarily responsive documents have been found.
(Grossman Decl., ¶ 10); see also Lawson v. Spirit AeroSystems, Inc., No. 18-1100, 2020 WL 1813395, at *7 (D. Kan. April 9, 2020). In addition, another metric relevant to this case is prevalence, also referred to as richness. This is the estimated proportion of responsive documents in a collection at the outset. For example, if there were 10 responsive documents in a set of 100 documents, the richness would be 10%. (Grossman Decl., ¶ 10).
Defendants’ Proposed Methodology
In the aggregate, the defendants have collected more than 10 million documents in response to the plaintiffs’ discovery demands. (Memorandum of Law in Support of Defendants’ Joint Motion for a Protective Order Regarding Certain Search Terms and TAR Methodologies (ECF 471) (“Def. Memo.”) at 1). Although there are minor differences among the methodologies suggested by each defendant, each fundamentally involves a five-step process for sifting through this information:
*3 1. The defendant would define the “TAR Review Set” by applying agreed search terms to its data collection and removing items not suitable for TAR (for example, video images or audio files).
2. The defendant would then take a random sample from the TAR Review Set and review it in order to obtain an estimate of the richness of the TAR Review Set, that is, the prevalence of responsive documents. The sample would be designed to achieve a 95% confidence level with a margin of error of 2%. For example, if the reviewers identified 72 responsive documents in a sample of 2,400 documents randomly selected from the TAR Review Set, it would be estimated that the richness of the entire TAR Review Set was 3%.
3. Next, the defendant would train the TAR predictive tool by “showing” it responsive (and, depending on the tool, non-responsive) documents. The tool would then score the documents within the TAR Review Set according to the likelihood that they are responsive. Because each defendant has indicated that it would use a continuous active learning tool, the determinations with respect to the documents reviewed would then be “fed back” to the classifier, refining its training and allowing it to rescore the documents remaining to be reviewed.
4. The defendant would continue the review process until it estimated that it had achieved a recall rate of 70%. This would be based on a calculation made from the previous estimation of richness. Thus, if the TAR Review Set were 100,000 documents, and the richness estimate was 3%, it would be expected that there would be approximately 3,000 responsive documents to be found. The defendant would pause its review when 70%, or 2,100 responsive documents, had been identified.
5. Finally, the defendant would validate its review by using an elusion test to determine if the 70% recall target had in fact been achieved. This would first involve calculating an estimate of the number of responsive documents that remained in the TAR Review Set and had not been reviewed (often referred to as the “null set”). See City of Rockford v. Mallinckrodt ARD, Inc., 326 F.R.D. 489, 493-94 (N.D. Ill. 2018) (describing sampling of null set in context of search terms). As with the initial richness estimate, this would be done by drawing a sample from the null set with a confidence level of 95% and a margin of error of 2%. Reviewers would then analyze this sample to determine the percentage of responsive documents and extrapolate from that the estimated number of responsive documents remaining in the null set. For example, if 70,000 documents had been reviewed from the corpus of 100,000 documents, this would leave 30,000 unreviewed documents in the null set. If a sample from that null set showed that 5% of those documents were responsive, it would be inferred that approximately 1,500 responsive documents (i.e., 30,000 × .05) remained in the null set had never been reviewed and had therefore “eluded” detection. From this, the defendant would then calculate the recall rate by dividing the number of responsive documents identified in the review (“R”) by the sum of the identified responsive documents (“R”) plus the estimate of eluded documents (“E”):
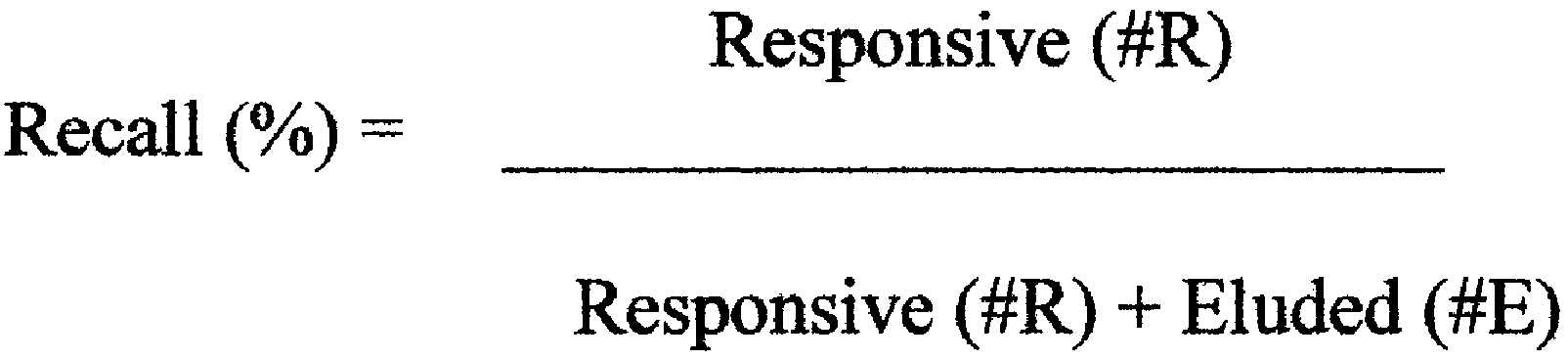
*4 In the example above, the number of responsive documents found was 2,100, and the number of eluded documents was 1,500. Therefore, the recall rate is:
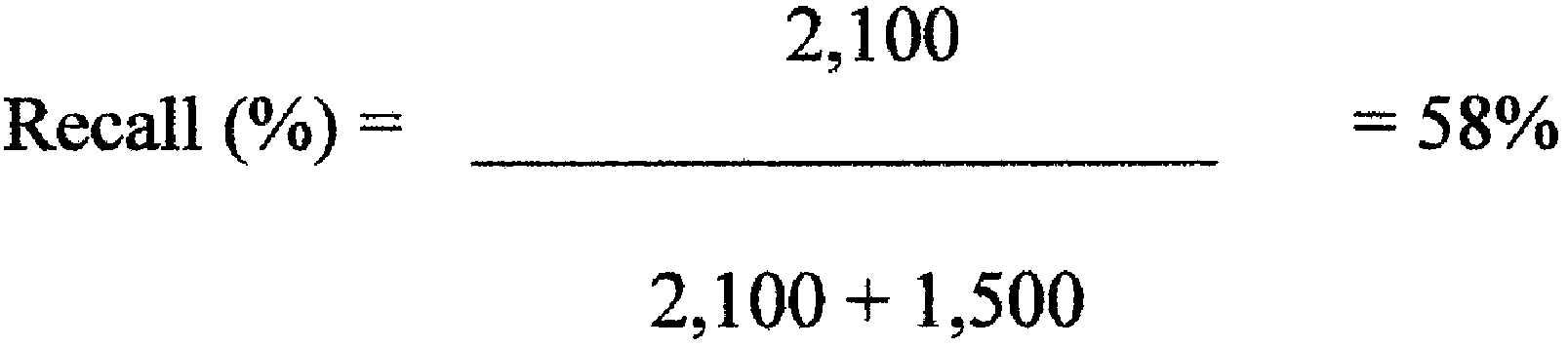
Since, in this example, the estimated recall rate fell below 70%, the defendant would resume its review process, coding additional batches of documents offered up by the TAR tool, until the 70% threshold had been reached, at which point it would halt its review. (Regard Decl., ¶¶ 31, 35).[2]
Plaintiffs’ Proposed Methodology
The plaintiffs’ proposal focuses on two aspects of the TAR workflow: the TAR review stopping criteria and the validation protocol.
The stopping criteria define the point at which a defendant pauses the review process. As discussed above, the defendants would do so when the number of responsive documents identified surpasses the 70% recall estimate, as indicated by reference to the estimated richness of the TAR Review Set. The plaintiffs’ proposal, by contrast, does not rely on an ex ante estimate of the richness of the entire TAR Review Set, but instead depends both on the richness of the last few batches of documents reviewed as well as the content of any responsive documents found in those batches.
The TAR process for each rolling production will continue until Defendants can reasonably conclude that further review is unlikely to yield additional responsive documents with sufficient quantity or materiality to justify continuing. This will not occur before the last two batches of documents identified by TAR and reviewed by humans contains no more than five to ten present (5%-10%) responsive documents, and none of the responsive documents is novel and/or more than marginally relevant.
(Plaintiffs’ Proposed Stopping Criterion and Validation Process for Defendants’ Application of Technology Assisted Review (“Pl. Proposal”) (ECF 460-3) at ECF 2). To make this work, the plaintiffs’ proposal requires each defendant to identify which responsive documents came from the last two batches and to reveal the number of documents from those batches deemed responsive but withheld because of privilege. If, on the basis of this information, the parties disagree whether the defendant chose an appropriate stopping point, the proposal requires them to meet and confer and, if no accommodation is reached, to present the dispute to the court. (PL Proposal at ECF 2).
The plaintiffs’ proposed validation protocol is complex, and is triggered only when, on the basis of the stopping criteria, the defendants “reasonably believe that they have produced or identified for production substantially all responsive non-privileged documents.” (Pl. Proposal at ECF 2).
The first step of the validation is the partition of the Tar Review Set into four Subcollections, from each of which a sample would be drawn as follows:
*5 • Subcollection 1: Documents identified by the review as responsive, including privileged documents, from which a random sample of 500 documents would be drawn.
• Subcollection 2: Documents coded as non-responsive by a human reviewer, from which a random sample of 1,500 documents would be drawn.
• Subcollection 3: Documents excluded from review because TAR assigned them a low probability of responsiveness, from which a random sample of 1,500 documents would be drawn.
• Subcollection 4: Documents excluded from review as a result of the application of search terms prior to the application of TAR, from which a random sample of 1,500 documents would be drawn.
(Pl. Proposal at ECF 3).
Next, each of the subsamples would be combined into a single Validation Sample of 5,000 documents that would be presented to one or more Subject Matter Experts (“SMEs”) who would, without knowledge of any prior coding determinations or of the subcollection from which any document was drawn, code each document as responsive or non-responsive. (Pl. Proposal at ECF 3). On the basis of the SME(s)’ determinations, the following calculations would then be made:
• Number of responsive documents found ≈ size of Subcollection 1 × number of responsive docs in the sample from Subcollection 1 ÷ 500.
• Number of responsive documents coded incorrectly ≈ size of Subcollection 2 × number of responsive documents in the sample from Subcollection 2 ÷ 1,500.
• Number of responsive documents excluded by TAR and not reviewed ≈ size of Subcollection 3 × number of responsive documents in Subcollection 3 ÷ 1,500.
• Number of responsive documents in the set of documents excluded by search terms ≈ size of Subcollection 4 × number of responsive documents from the sample of Subcollection 4 ÷ 1,500.
(Pl. Proposal (Appendix A) at ECF 5). These defined terms would then be combined to calculate estimated recall as follows:
Estimated recall ≈ Number of responsive documents found ÷ (number of responsive documents found + number of responsive documents coded incorrectly + number of responsive documents excluded by TAR and not reviewed + number of responsive documents in the set of documents excluded by search terms).
(Pl. Proposal (Appendix A) at ECF 5). Under the plaintiffs’ proposal, while the “estimate of recall should be computed to inform the decision-making process” with respect to when a defendant may stop reviewing, “the absolute number in its own right shall not be dispositive of whether or not a review is substantially complete.” (Pl. Proposal (Appendix A) at ECF 5). Rather, the parties would also consider the novelty and materiality of documents now deemed responsive that had previously been coded as non-responsive or had been excluded from review by either search terms or the TAR tool. (Pl. Proposal (Appendix A) at ECF 5).
At this point it is useful to highlight the differences between the defendants’ and the plaintiffs’ recall estimation processes, an issue that will be revisited later. In broad terms, the formulas are similar in that each is the ratio between the number of responsive documents found and the sum of the number of responsive documents plus the number of responsive documents that were missed. There are, however, important differences. The second term in the denominator of the plaintiffs’ formula – the number of responsive documents coded incorrectly – does not appear in the defendants’ calculations. Thus, the plaintiffs attempt to account for reviewer error, whereas the defendants do not. Furthermore, the fourth term in denominator of the plaintiffs’ formula – the number of responsive documents in the set of documents excluded by search terms – likewise is omitted from the defendants’ formula. Accordingly, while the plaintiffs would calculate recall for both the search term and the TAR segments of the review, the defendants would calculate it only for the TAR portion.[3]
Governing Legal Principles
*6 Rule 26(g) requires that a party responding to discovery requests must conduct “a reasonable inquiry.” Particularly in the realm of electronic discovery, where the volumes of data can be vast and the costs of locating every responsive document can be enormous, this obligation does not require perfection. See Lawson v. Spirit AeroSystems, Inc., No. 18-1100, 2020 WL 1813395, at *7 (D. Kan. April 9, 2020) (“[W]hile parties must impose a reasonable construction on discovery requests and conduct a reasonable search when responding to the requests, the Federal Rules do not demand perfection.” (citation omitted)); Winfield v. City of New York, No. 15-CV-05236, 2017 WL 5664852, at *9 (S.D.N.Y. Nov. 27, 2017) (“[P]erfection in ESI discovery is not required; rather, a producing party must take reasonable steps to identify and produce relevant documents”); Hyles v. New York City, No. 10 Civ. 3119, 2016 WL 4077114, at *3 (S.D.N.Y. Aug. 1, 2016) (“[T]he standard is not perfection, or using the “best” tool, but whether the search results are reasonable and proportional.” (citation omitted)); Enslin v. Coca-Cola Co., No. 2:14-cv-06476, 2016 WL 7042206, at *3 (E.D. Pa. June 8, 2016).
As applied to the complexities of TAR, the principle of reasonableness incorporates an obligation for the producing party to validate its search. While it might be appropriate in a simple case to assume that a properly instructed custodian can conduct a reasonable search of a paper file without the need for a post hoc evaluation of the custodian's search process, the same cannot be said for a technology-assisted search of hundreds of thousands of documents. Accordingly, courts have required parties to validate their TAR search methodologies. See Mercedes-Benz Emissions Litigation, No. 2:16-CV-881, 2020 WL 103975, at *2 (D.N.J. Jan. 9, 2020) (finding that “case law dictates that appropriate validation be utilized to test search results”); City of Rockford, 326 F.R.D. at 494 (holding that “validation and quality assurance are fundamental principles to ESI production”). This requirement for validation applies to search terms just as it does to TAR. See, e.g., In re Seroquel Products Liability Litigation, 244 F.R.D. 650, 662 (M.D. Fla. 2007) (“[W]hile key word searching is a recognized method to winnow relevant documents from large repositories, use of this technique must be a cooperative and informed process.... Common sense dictates that sampling and other quality assurance techniques must be employed to meet requirements of completeness.”).
A further relevant legal principle is that “a producing party has the right in the first instance to decide how it will produce its documents.” In re Valsartan, 337 F.R.D. at 617; see also Winfield, 2017 WL 5664852, at *9; Hyles, 2016 WL 4077114, at *2. This general rule does not, however, give carte blanche to a producing party. Rather, “a producing party is best situated to determine its own search and collection methods so long as they are reasonable.” Nichols v. Noom, Inc., No. 20-CV-3677, 2021 WL 948646, at *2 (S.D.N.Y. March 11, 2021) (emphasis supplied). Thus, “ ‘[p]arties cannot be permitted to jeopardize the integrity of the discovery process by engaging in halfhearted and ineffective efforts to identify and produce relevant documents.’ ” Winfield, 2017 WL 5664852, at *9 (quoting HM Electronics, Inc. v. R.F. Technologies, Inc., No. 12-cv-2884, 2015 WL 471498, at *12 (S.D. Cal. Aug. 7, 2015), vacated in part on other grounds, 171 F. Supp. 3d 1020 (S.D. Cal. 2016)). Moreover, the right of a producing party to choose a general search method – search terms rather than TAR, for example – does not mean that a court must blindly accept all of the specific details of the proffered methodology. For instance, a party that could legitimately to use search terms as its preferred method could not then specify terms that would exclude large swaths of relevant documents. Thus, the decisions holding merely that a court should defer to the producing party's choice to use a generic search methodology, see, e.g., In re Viagra (Sildenafil Citrate) Products Liability Litigation, No. 16-md-02691, 2016 WL 7336411, at *1-2 (N.D. Cal. Oct. 14, 2016) (refusing to compel use of predictive coding); Hyles, 2016 WL 4077114 at *3 (finding that “it is not up to the Court, or the requesting party ..., to force the ... responding party to use TAR when it prefers to use keyword searching”), provide little guidance in a case like this, where the defendants’ decision to use TAR is not in dispute.
*7 Discovery is also informed by the related, but not identical, concepts of cooperation and transparency. “Technology-assisted review of ESI ... require[s] an ‘unprecedented degree of transparency and cooperation among counsel.’ ” Youngevity International Corp. v. Smith, No. 16-cv-00704, 2019 WL 1542300, at *12 (S.D. Cal. April 9, 2019) (quoting Progressive Casualty Insurance Co. v. Delaney, No. 2:11-CV-00678, 2014 WL 3563467, at *10 (D. Nev. July 18, 2014)); accord In re Valsartan, 337 F.R.D. at 622. The principle of cooperation is not only established in the case law, it is mandated here both by the Court's order governing discovery of ESI in this case (Stipulated Order re: Discovery of Electronically Stored Information dated April 27, 2020 (ECF 313) (“ESI Order”), ¶ 2) and by the Local Rules for the Western District of Pennsylvania (Appendix LCvR 26.2C, Guideline 1.02). However, by itself the requirement to cooperate only goes so far. Cooperation requires good faith efforts to reach agreement on material issues, but it does not require that agreement always be reached. Parties may fulfill their cooperation obligations without resolving all disputes between them.
Transparency transcends cooperation. It does not mean merely that parties must discuss issues concerning the discovery of ESI; it requires that they disclose information sufficient to make those discussions, as well as any court review, meaningful. In this case, the parties agreed, and the Court ordered that “[i]f a party decides to use a computer/technology assisted review (“TAR”) for identifying potentially responsive documents, it shall inform the other parties and, if requested, shall discuss methods and procedures for implementing it.” (ESI Order, ¶ 5(a)(i)). Transparency, then, may go beyond simply identifying the nature of the search methodology to be used. See Rio Tinto PLC v. Vale S.A., 306 F.R.D. 125, 128 (S.D.N.Y. 2015) (declining to rule on seed set transparency where parties agreed to disclose all non-privileged documents in control sets); Bridgestone Americas, Inc. v. International Business Machines Corp., No. 3:13-1196, 2014 WL 4923014, at *1 (M.D. Term. July 22, 2014) (approving party's agreement to reveal seed set used to train TAR); In re Actos Products Liability Litigation, No. 6:11-md-299, 2012 WL 7861249, at *4-5 (W.D. La. July 27, 2012) (noting that experts for each party reviewed and coded TAR seed set pursuant to parties’ ESI protocol). As the court observed in Winfield, “[c]ourts are split as to the degree of transparency required by the producing party as to its predictive coding process.” Winfield, 2017 WL 5664852, at *10.
The procedural mechanisms that the parties rely on here in seeking the Court's intervention are a motion to compel discovery under Rules 26 and 37 and a motion for a protective order under Rule 26(c). Pursuant to Rule 37(a)(3)(B)(iv), “[a] party seeking discovery may move for an order compelling ... production ... if ... a party fails to produce documents or fails to respond that inspection will be permitted – or fails to permit inspection – as requested under Rule 34.” The scope of discovery, in turn, is defined by Rule 26.
Unless otherwise limited by court order, the scope of discovery is as follows: Parties may obtain discovery regarding any nonprivileged matter that is relevant to any party's claim or defense and proportional to the needs of the case, considering the importance of the issues at stake in the action, the amount in controversy, the parties’ relative access to relevant information, the parties’ resources, the importance of the discovery in resolving the issues, and whether the burden or expense of the proposed discovery outweighs its likely benefit. Information within this scope of discovery need not be admissible in evidence to be discoverable.
Fed. R. Civ. P. 26(b)(1). See generally Southeastern Pennsylvania Transportation Authority v. Orrstown Financial Services, Inc., 367 F. Supp. 3d 267, 276 (M.D. Pa. 2019); Focht v. Nationstar Mortgage, LLC, No. 3:18-cv-151, 2019 WL 3081625, at *1 (W.D. Pa. July 15, 2019).
*8 Under Rule 26(c), a “court may, for good cause, issue an order to protect a party or person from annoyance, embarrassment, oppression, or undue burden or expense.” Fed. R. Civ. P. 26(c)(1). The moving party bears the burden of demonstrating good cause, and must do so by showing that disclosure will result in clearly defined specific and serious injury; broad allegations of harm are insufficient. Shingara v. Skiles, 420 F.3d 301, 306 (3d Cir. 2005). In determining whether good cause exists, “the court must balance the need for information against the injury that might result if uncontrolled disclosure is compelled.” Engage Healthcare Communications, LLC v. Intellisphere, LLC, No. 12-cv-00787, 2016 WL 11680950, at *2 (D.N.J. Nov. 30, 2016) (quotation marks and citation omitted).
I will now address the merits of the parties’ motions. Because the defendants’ production obligations are at issue, I will begin with their motion.
Defendants’ Motion for a Protective Order
A. TAR Methodology
The defendants essentially seek the Court's blessing for their search terms and TAR methodologies in advance of having conducted any review of the bulk of their documents. Assuming that this is an appropriate subject for a protective order in the first place, the motion should be denied because the proposed methodologies contain serious flaws that would preclude the defendants from certifying their discovery responses to be reasonable under Rule 26(g).
First, the defendants’ validation protocol is defective because it is limited to the TAR portion of the search and review process. As the defendants recognize, selecting documents for production involves a succession of independent steps, each of which is designed to help identify responsive information and exclude non-responsive data. (Tr. at 21). Initially, documents are collected from the entire universe of a defendant's data, by the identification of custodians and other specific data sources. Next, as in this case, search terms may be applied to filter the information further. The remaining documents become the TAR Review Set to which the TAR algorithm is applied. The documents recommended by the TAR tool as most likely responsive are then analyzed by human reviewers who make a final determination of responsiveness before the documents are produced. At each of these stages except the last, responsive documents may be left behind and never reviewed by humans, and at the final stage, responsive documents may be mistakenly coded as non-responsive.
In this case, the parties have not disputed the defendants’ initial collection processes. And they have generally agreed that 70-80% estimated recall is often considered reasonable by the courts. (Letter of Sarah R. LaFreniere dated March 4, 2021 (ECF 458-8) (“LaFreniere 3/4/21 Ltr.”), attached as Exh. G to Declaration of Sarah R. LaFreniere dated March 25, 2021 (ECF 458), at 1). But what that recall figure is to be derived from is a matter of dispute. The defendants suggest that the parties agreed that 70% recall based on search and review only of the Tar Review Set would be deemed reasonable. (Def. Memo. at 14). Not so. First, any such agreement would have to be predicated on an agreement as to what constitutes the TAR Review Set, something that has not been achieved since the parties have not agreed on the search terms that would define that set. Second, even had there been such an agreement, it is apparent that the plaintiffs anticipated estimating recall based on each step of the process following the initial collection, as illustrated by the stratified sampling method that they proposed which included documents eliminated from consideration by search terms. (LaFreniere 3/4/21 Ltr. at 5-6; see also Letter of Hallie E. Spraggins dated Feb. 19, 2021 (ECF 458-6), attached as Exh. E to LaFreniere 3/25/21 Decl., at 4-6 & App. A; Letter of Alden L. Atkins dated March 16, 2021 (ECF 458-9), attached as Exh. H to LaFreniere 3/25/21 Decl., at 6). There was thus no meeting of the minds as to the segments of the search and review process that would be included in calculation of the recall estimate.
*9 In the absence of such agreement, it would be plainly unreasonable to calculate estimated recall for the TAR portion of the process alone. Dr. Maura R. Grossman, the plaintiffs’ expert, provides an example showing why this is the case. Suppose a party collected one million documents, of which 100,000 are responsive. If search terms cull out 600,000 documents, then 400,000 become the TAR Review Set. Suppose further that of the 400,000 documents to which TAR is applied, 70,000 are responsive. That means that the search term portion of the workflow would have left 30,000 responsive documents behind, and the estimated recall for that stage would be 70% (that is, 70,000 ÷ (70,000 + 30,000)). Then assume that the TAR review – the only part of the process that the defendants propose to validate – reached their target of 70% recall because 49,000 responsive documents were identified (49,000 ÷ (49,000 + 21,000) = 70%). The overall estimated recall for the search term and TAR stages would then be the product of the recall for each stage: 49% (70% × 70%, or, calculated as “end-to-end recall, 49,000 ÷ (49,000 + 51,000)). (Affidavit of Maura R. Grossman dated March 25, 2021 (ECF 459), ¶ 37). In other words, a defendant would claim a recall rate of 70% when, in fact, it had produced less than half of the responsive documents.
The second flaw in the defendants’ protocol is its failure to take advantage of the specific characteristics of CAL that provide the parties a means for predicting not just the quantity, but also the quality, of likely responsive documents that have not yet been reviewed by humans. When parties have utilized either search terms exclusively or earlier versions of TAR, courts have occasionally required that the results of their searches be validated in part by allowing the requesting party to review a sample of the “null set,” that is, the set of documents deemed non-responsive by the search device and not subjected to human review. See City of Rockford, 326 F.R.D. at 493-95 (review of sample of documents excluded by search terms); Winfield, 2017 WL 5664852, at *11 (review of sample of documents excluded by TAR). Some courts are understandably reluctant to require follow this course, at least in the absence of a showing of some deficiency in the production, because it seems contrary to the rules of discovery to require disclosure of non-responsive documents.
CAL provides a means for achieving greater confidence in the completeness of a search without the need to reveal non-responsive documents. Because the CAL tool is continuously being retrained and is reranking as yet unreviewed documents in response to what it “learns” from the coding of documents that have been reviewed by humans, the number of likely responsive documents in each successive batch to which the tool is applied diminishes steadily after the algorithm has been sufficiently trained. As Mr. Regard, the defendants’ expert, has attested, “[i]n at TAR review, once the TAR system is trained, a large proportion of the documents batched for review in the early stages are responsive. As the review continues, the proportion of responsive documents declines, often significantly.” (Regard Decl., ¶ 19). Thus, by looking at the number of responsive documents in the last couple of batches analyzed by the tool (or, more precisely, by looking at the percentage of responsive documents out of the total number of documents reviewed in each of those batches), a party can evaluate the trend and predict the estimated number of likely responsive documents in the next batch. Perhaps more importantly, a party can look at the content of the responsive documents identified from the last couple of batches to assess their significance and thereby predict whether documents in the next batch would likely be not merely responsive, but important to the litigation. For example, suppose the last batches of documents contain invoices which, although technically responsive, are of little import to the outcome of the case. In that instance, there would be little reason to continue the search. On the other hand, if those batches contain “hot” documents, searching additional batches would be prudent.
The defendants argue that because parties commonly rely on recall as the sole basis for validation, their approach is reasonable. But what constitutes reasonable conduct must necessarily be measured against the available technology. Using validation based exclusively on elusion testing and recall statistics may be reasonable for parties using only search terms or TAR 1.0. But CAL gives the parties a powerful method for evaluating search at the margin, helping them decide whether further search and review will be proportional. For the defendants to have this tool in their hands but to forgo its use is unreasonable.
*10 Because the defendants’ proposed TAR procedures include a validation process that is fundamentally flawed, their motion for a protective order permitting them to utilize those procedures should be denied.
B. Search Terms
The defendants further seek a protective order permitting them to utilize their designated search terms to cull their documents prior to the application of TAR. The parties agreed that search terms may be used for this purpose. (Declaration of Zachary K. Warren dated April 9, 2021 (ECF 472) (“Warren Decl.”), Exh. 1; Pl. Memo at 1). They also agreed to a procedure for collaborating on and, if necessary, obtaining a ruling on disputed search terms. (ESI Order, ¶ 5(a)(2)). That process has broken down.
At the point where the parties reached impasse, the plaintiffs’ suggested search strings returned between 67% and 87% of the documents collected, depending upon defendant, while the hit rate for the defendants’ suggested search strings ranged from 29% to 55%:
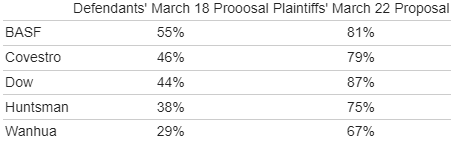
(Warren Decl., ¶ 24). The defendants argue that if they are not permitted to use their search terms and are instead required to use the plaintiffs’ terms, they will be forced to review large numbers of irrelevant documents at disproportionate cost and burden.
Yet the defendants have done no systematic testing of the proposed terms to determine whether their search strings leave behind significant numbers of responsive documents captured by the plaintiffs’ terms. This has two consequences. First, without such testing, the defendants cannot represent that their proposed search terms are substantively reasonable. As the defendants’ expert has written,
[t]ypically, in a modern, iterative development process, false negatives are examined to understand why a set of search terms might be missing a known responsive document. This technique, when available, can be used to identify new query strings that will add to the overall recall of a set of search terms. This is often used to calibrate search terms and can lead to recall improvement.
(Dan Regard & Tom Matzen, A Re-Examination of Blair & Maron (1985), ICAL 2013 DESI V Workshop, Position Paper at 10-11 (June 14, 2013), available at https://docplayer.net/2626527-A-re-examination-of-blair-maron-1985.html). Second, without testing it is impossible to make a proportionality assessment, since the incremental value of the additional search terms proposed by the plaintiffs remains unknown. As will be discussed further below, the defendants did conduct some testing of their search terms against a portion of their collection: documents that they produced in the context of a Department of Justice investigation. (Declaration of Trisha Jhunjhnuwala dated April 30, 2021 (ECF 496, ECF 497 (sealed)) (“Jhunjhnuwala Decl.”), ¶ 13). But the results of testing a collection of documents already selected as responsive cannot be generalized to the far broader collection to which the defendants would apply their search terms in order to create the TAR Review Set.
Furthermore, the large percentage of documents collected by the plaintiffs’ search terms, standing alone, is not meaningful. In discussing search terms that retrieved 80 percent of an office's emails, the District of Columbia Court of Appeals observed that, “far from showing bad faith, that figure may simply indicate that most of the emails actually bear some relevance, or at least include language captured by reasonable search terms.” In re Fannie Mae Securities Litigation, 552 F.3d 814, 821 (D.C. Cir. 2009). In this regard, it should be kept in mind that the function of search terms in this case is not to identify documents for production or even to select those that will be provided directly to human reviewers; it is to narrow the universe of documents to which TAR will be applied. In this context, precision, which is what the defendants appear to seek, is relatively less important than recall.[4]
*11 The defendants’ focus on hit rates without regard for the substance of documents excluded by search terms is particularly problematic given the nature of this case. “The choice of a specific search and retrieval method will be highly dependent on the specific legal context in which it is to be employed. Parties and their counsel must match the use case with the tools and best practices appropriate to address it....” The Sedona Conference Best Practices Commentary on the Use of Search and Information Retrieval Methods in E-Discovery, 15 Sedona Conf. J. 217, 244 (2014). Here, the plaintiffs’ allegations of an antitrust conspiracy suggest that broader search terms may be warranted than in a case where pertinent communications might be less guarded. One specific dispute illustrates this. The defendants contend that the plaintiffs’ search terms are overly general: “[t]he term ‘in person,’ for example, is only relevant as it relates to a meeting with a competitor, so it should be limited to documents that include a competitor's name.” (Def. Memo. at 19). But in everyday communications, let alone communications that might lead to antitrust liability, a person is at least as likely to say, “I'm having an in person meeting with Joe” as they are to say, “I'm having an in person meeting with Covestro.” Yet the defendants’ narrower approach would preclude documents like the hypothetical email about the meeting with Joe from even reaching the stage of TAR review.
The defendants have not only failed to show that their suggested search terms are reasonable, they have also failed to demonstrate that using more expansive terms, even if not those suggested by the plaintiffs, will create an unacceptable burden. Some of this appears to be related to a misunderstanding of the relation between the search terms and the TAR review. For example, in rejecting proposed terms that the defendants consider too generic, they contend that they “should not be required to review every document relating to lunch prices or meeting minutes.” (Def. Memo at 19). But because the defendants are not proposing to do a full manual review following the application of search terms, there is no need to review “every document.” Rather, since the CAL tool ranks documents according to responsiveness, documents relating to lunch prices or meeting minutes that do not also have some other indicator of relevance would soon be ranked near the bottom of the Tar Review Set and would likely never be assessed by a human reviewer.
The defendants’ TAR vendors make somewhat more subtle arguments regarding burden. First, they contend that the more documents that are included in the TAR Review Set, the higher the hosting costs. (Declaration of Tony Reichenberger dated April 9, 2021 (ECF 471-6), ¶ 9; Declaration of Joseph Goodman dated April 9, 2021 (ECF 471-4), ¶ 6); see also Lawson v. Spirit AeroSystems, Inc., No. 18-1100, 2020 WL 3288058, at *19 (June 18, 2020) (finding that “the volume of data subjected to the TAR process materially impacts technology costs such as data processing and hosting”). Second, they argue that because the additional documents included if the plaintiffs’ search terms are used would reduce the richness of the TAR Review Set while also potentially adding novel concepts and issues, it would be harder to train the CAL algorithms. In other words, more human review would be required at the front end before the tools begin returning a high proportion of responsive documents. (Shebest Decl., ¶ 10); Goodman Decl., ¶ 7; Declaration of Xavier P. Diokno dated April 9, 2021 (ECF 471-5), ¶ 26); see also Lawson, 2020 WL 3288058, at *19 (finding that “[w]hen ‘the TAR set's richness is extremely low, human reviewers may have a difficult time training the software on what is relevant, because examples may be scarce or difficult to come by in the TAR set’ ” (quoting Duke TAR Guidelines at 27)).
These points are well taken. However, the defendants’ efforts to quantify this burden are less successful. Although hosting costs could presumably be calculated simply on the basis of the volume of additional documents captured by the plaintiffs’ search terms, the defendants have not specified those costs. Some defendants have attempted to estimate additional review costs by making the assumption that to achieve 70% recall, approximately 75% of the documents would need to be reviewed. They then project review costs by applying this 75% figure to the number of additional documents collected by the plaintiffs’ search terms. (Shebest Decl, ¶¶ 11-13). But the defendants acknowledge that the 75% figure “will vary for each set of documents and each case.” (Shebest Decl., ¶ 11). Moreover, Dr. Grossman vigorously disputes the 75% assumption, pointing to academic studies suggesting that, on average, parties that use CAL review only one to two non-responsive documents for each responsive document. (Supplemental Declaration of Marua R. Grossman dated April 30, 2021, ¶¶ 33-35, 70).
*12 Thus, the defendants have not provided sufficiently reliable cost estimates to justify the wholesale rejection of the plaintiffs’ search terms, nor have they shown that their own search terms will capture a reasonable proportion of the responsive documents. Accordingly, the defendants’ motion for a protective order allowing them to use their suggested search terms should be denied.
Plaintiffs’ Motion to Compel
That the defendants’ motion should be denied does not, however, mean that the plaintiffs’ motion to compel should be granted. The principle that the producing party is the master of its methodology is a deterrent to imposing a requesting party's proposed procedures unless it is evident that the producing party is unable to come up with a reasonable alternative. That is not the case here. While the defendants’ search term and TAR methodologies are not reasonable, the plaintiffs’ proposals go beyond what the law requires in at least some respects.
A. TAR Methodology
The plaintiffs contend that the defendants should be required to continue running their CAL tools until two sequential batches contain less than 5-10% responsive documents and no responsive documents that are novel or more than marginally relevant. As discussed above, when the search process is on the brink of completion, it is critical for the parties to give attention both to the richness of the last batches and to the significance of documents found in those batches. However, it would be arbitrary to impose rigid stopping criteria. For example, if more than 10% of the documents in the last few batches were technically responsive, it might nevertheless be reasonable to stop if, as in the example cited earlier, those responsive documents were all invoices of marginal value to the litigation. Furthermore, to the extent that the plaintiffs suggest that the TAR methodology must identify “substantially all responsive non-privileged documents” in order to be valid, they misstate the legal standard, which requires reasonableness, not perfection.
Similarly, the plaintiffs’ validation criteria overreach by incorporating an analysis of the accuracy of the determinations made by human reviewers. There is no doubt that reviewers often come to differing conclusions regarding the responsiveness of the same documents. See, e.g., Marua R. Grossman & Gordon V. Cormack, Technology-Assisted Review in E-Discovery Can Be More Effective and More Efficient Than Exhaustive Manual Review, XVII Rich. J.L. & Tech. 1, 10-13 (2011). And there is also no doubt that the determinations of reviewers are often wrong when judged against the determinations of a subject matter expert, as on a second-level review. Id. at 13-14. This is true whether the reviewers are engaged in a linear review of all documents collected or in a review of documents culled by search terms or by TAR. Yet courts have generally not mandated the incorporation of such reviewer error in calculating the recall statistic in any of these scenarios. This may be due, in part, to the fact that by the time recall is being calculated, the ability to correct for reviewer error has long since passed. Responsible producing parties instead monitor reviewer performance on an ongoing basis, so that a reviewer who is making erroneous responsiveness determinations can be retrained or replaced and the determinations they have already made can be audited.
*13 It is true, as the plaintiffs argue, that failure to account for reviewer error will artificially inflate the recall statistic because that statistic will include non-responsive documents incorrectly coded as responsive. (Grossman Decl., ¶ 37). But what is deemed an acceptable recall estimate can be adjusted to take human error into consideration. For example, if 70% recall were considered reasonable if human error were included in the calculation, perhaps 72% or 75% or some other percentage would be reasonable if human error is not taken into account.
Thus, since there are alternative TAR methodologies that the defendants could utilize and that are reasonable, they should not be compelled to adopt the plaintiffs’.
B. Search Terms
Similarly, whatever the flaws in the defendants’ search terms, the plaintiffs have failed to demonstrate that their proposed search terms are the only reasonable option. For example, the plaintiffs applied their disputed search terms to the documents that the defendants had produced to the Department of Justice and found that these terms resulted in the identification of many arguably responsive documents. (Pl. Memo. at 2-3 & Exh. A). From this, they argue that use of the defendants’ terms would have excluded these documents from the TAR Review Set altogether. But that is not the case. The defendants have demonstrated that, with one exception, every one of the exemplary documents cited by the plaintiffs would have been elicited by the defendants’ proposed search terms. (Jhunjhnuwala Decl., ¶¶ 19-20). The single exception is a document that does not appear to be responsive to the plaintiffs’ document requests (Jhunjhnuwala Decl., ¶ 21), so its exclusion from the TAR Review Set would not prejudice the plaintiffs.
Thus, while the plaintiffs’ search terms (or, indeed, the use of no search terms at all) may be a reasonable means of creating the TAR Review Set, there is no basis for precluding the defendants from utilizing a different set of search terms that is also reasonable.[5] Accordingly, insofar as it relates to search terms, the plaintiffs’ motion to compel should be denied.
Conclusion
For the reasons set forth above, I respectfully recommend that Plaintiffs’ Motion to Compel Defendants to Use Certain Search Terms and TAR Methodologies (ECF 455) and Defendants’ Joint Motion for a Protective Order Regarding Certain Search Terms and TAR Methodologies (ECF 470) each be denied.
Footnotes
This group does not include additional defendants who are not party to these motions.
Although the description of the defendants’ methodology, including the formulas, is derived from the declaration of their primary expert, Daniel L. Regard II, I have provided the numerical example for clarity, and any arithmetic errors are my own.
At oral argument, the defendants suggested for the first time that they, in fact, intended to validate their search by calculating an estimate of recall that took into account documents excluded both by search terms and by TAR (Transcript of Oral Argument dated Aug. 2, 2021 (“Tr.”) at 68-69). That would be a marked change in position. The defendants’ stated validation process defines the “TAR Review Set” as the documents identified after application of search terms and then calculates recall on the basis of documents remaining in the TAR Review Set that had not been subject to review. (Regard Decl., ¶ 31). Moreover, the defendants criticized the plaintiffs’ proposed validation method precisely because it incorporates the search term phase. (Defendants’ Joint Memorandum of Law in Opposition to Plaintiffs’ Motion to Compel Defendants to Use Certain Search Terms and TAR Methodologies (“Def. Opp. Memo.”) (ECF 492) at 7; Declaration of Stephen Shebest in Support of Defendants’ Motion for a Protective Order dated April 8, 2021 (“Shebest Decl.”) (ECF 471-3), ¶ 18).
Precision remains significant to the extent that it affects the cost of implementing TAR, as will be discussed below.
The ESI Order provides a mechanism for adjudicating disputes over search terms. (ESI Order, ¶ 5(a)(2)). If need be, the parties can seek a court decision with respect to their disagreements over individual terms.